Abstract
A major health policy concern is the presence of inefficiencies in health care provision. This study estimates the technical efficiency for ambulatory services and inpatients care in private sector hospitals in Pakistan. Efficiency scores for the sample hospitals, estimated using Stochastic Frontier Analysis, are aggregated at the regional (district) level to identify the existence of spatial interdependence. The results from the spatial analysis suggest that efficiency has a positive spillover for outpatient care in small hospitals. Big hospitals, however, show inconsistent results. We concluded that small hospitals compete in outpatients with the motive of profit maximization.
Key Words
Private Hospitals, Efficiency, SFA, Spatial Dependence, Competition, Pakistan
Introduction
In the past few years, healthcare expenditures have been increasing significantly not only in the absolute term but also as a proportion of GDP (Committee, 2012).To lessen this financial burden, governments in many western countries have initiated pro-competition reforms in the hospital sector. The United Kingdom, the United States, and the Netherlands were the first to introduce competition in the hospital market. Subsequently, other countries also started encouraging competition in their hospital market to provide a better choice to the patients. The existing empirical evidence on the real effects of pro-competition reforms on health outcomes is, nonetheless, mixed. Some studies conclude that competition leads to better health outcomes (Gaynor, Propper, & Seiler, 2016); others claim that increase in competition may worsen the peoples’ health conditions (Propper, Burgess, & Green, 2004); whereas others (Berta, Martini, Moscone, & Vittadini, 2016; Colla, Bynum, Austin, & Skinner, 2016; Mukamel, Zwanziger, & Bamezai, 2002) suggest that there is no relationship between competition and quality.
These empirical studies assumed that hospitals compete within the local market in a pre-specified geographical area to attract more patients. However, according to some studies (Gravelle, Santos, & Siciliani, 2014), hospitals have incentives to compete beyond their geographical boundaries. For instance, some empirical studies comprehensively analyze the efficiency, effectiveness and quality of healthcare providers using data at a geographic/administrative level considering the interdependence of hospitals of different regions (Augurzky & Schmitz, 2010; Felder & Tauchmann, 2009). When the aggregated regional level data is used, the assumption of independent observations used in conventional regression analysis becomes indefensible because the healthcare system in different regions cannot be separated. Ignoring the assumption of spatial interdependence due to patients’ mobility across regions of residence can bias the regional efficiency scores. According to Augurzky and Schmitz, less than 50 percent of total patients are treated within a region of residence in some areas (Augurzky & Schmitz, 2010).
The traditional approach was to investigate the effect of competition on efficiency and quality by using market concentration measures like the Herfindahl Index. Recently, however, researchers have started to use spatial analysis to examine strategic interactions among hospitals (Herwartz & Strumann, 2012; Francesco Longo, Siciliani, Moscelli, & Gravelle, 2017).
According to literature, hospitals’ negative spatial interdependence shows the existence of competition for low-cost patients. Although a hospital that is successful in attracting more patients from its rival shows better performance (Herwartz & Strumann, 2012), this can also be another way round, especially when a country has a mixed health care system (public and private) like Pakistan. Positive spillover of efficiency can also show the existence of competition. At the same time, both neighbouring regions can be successful in attracting more patients. There can be two potential reasons behind this behaviour, First, the presence of unattended patients who are not getting treatment from any hospital. Secondly, private sector hospitals may be attracting patients from the public sector (e.g. due to low-quality service or bad reputation, etc.), which can also be observed by the current expanding role of the private sector in Pakistan.
A deep understanding of the performance evaluation and spillover effects of the technical efficiency of private hospitals will be more effective in designing the policies and health interventions. Do private hospitals compete, in the form of efficiency spillovers, to attract more patients for profit maximization in a developing country like Pakistan? In other words, whether the hospitals of one district react to an increase or decrease in efficiency by their rivals in a competitive environment. To answer this question, the underlying study has examined the existence of strategic interactions among hospitals in the geographical area. That is, whether the technical efficiencies are strategic complement or substitute. Our analysis follows a two-step approach. First, the technical efficiency scores are estimated using the Stochastic Frontier Analysis (SFA). Subsequently, the spatial interdependence of private healthcare provision at the district level is analyzed for Pakistan.
Rest of the paper is organized as follows: the second section explains data, variables and econometric methodology. Results are discussed in third section and last section provides the concluding remarks, some policy suggestions and future research gap.
Data and Variables
The
private health care sector in Pakistan consists of small health care providers
(number of beds <50) and big private hospitals (number of beds >50). The
small health care providers consist of small hospitals, individually run general
practitioner clinics, dental clinics, specialty clinics, paramedics running
clinics, outpatient care centers, laboratories and diagnostic services,
homeopaths, hakeems, tabibs and other traditional health care providers. The
Pakistan Bureau of Statistics (PBS) conducted a performance evaluation survey
of 21,486 healthcare providers and a census of all 125 big hospitals in 2010-11
from the four provinces across Pakistan. The survey and census collected
information on the number of inpatients and outpatient treated in the previous
year, the existing number of doctors, specialists, and paramedical staff, other
staff, and the number of beds in the facility. For our analysis, we have taken
all the health care centers that provide the impatient facility. This includes
all the big hospitals as well as 624 small health care providers.
The number of inpatients and outpatients treated in the health
facility is used as output variables in the calculation of efficiency scores of
hospitals. These are the most widely
used measures of outcome in hospital efficiency studies (Valdmanis, Rosko,
& Mutter, 2008). On the other hand, for input variables, the study included
general practitioner doctors, specialist doctors, paramedical staff, other
staff, and the number of beds. These variables are commonly used in the
literature as representatives of productive factors, human resource and capital
(Navarro-Espigares & Torres, 2011). Efficiency measurement is all about
efficient resource utilization and the empirical evidence suggests that doctors
are accountable for 80 percent of hospitals’ resource utilization (Chilingerian
& Sherman, 1997). Hence, the physicians (general practitioner doctors and
specialist doctors) and paramedical staff are selected as a measure of human
resources (labor). Moreover, the number of beds is taken as a measure of
capital. The descriptive statistics of all the variables included in the
analysis are provided in Table 1.
Table
1. Descriptive Statistics of Variables
Variables |
Number of Obs. |
Mean |
Std. Deviation |
Minimum |
Maximum |
Outputs |
|
|
|
|
|
Inpatients |
749 |
1,800 |
6,809 |
1 |
144,497 |
Outpatients |
749 |
17,132 |
52,637 |
1 |
967,994 |
Inputs |
|
|
|
|
|
No
of beds |
749 |
34.49 |
78.66 |
2 |
747 |
Doctors |
749 |
7.348 |
23.55 |
1 |
323 |
Specialists |
749 |
6.742 |
15.96 |
1 |
200 |
Paramedical
Staff |
749 |
26.13 |
104.5 |
1 |
1,744 |
Other
Staff |
749 |
27.94 |
200.2 |
1 |
4,564 |
Control Variables |
|
|
|
|
|
Prenatal
Care |
80 |
64.42 |
13.92 |
32 |
94 |
Immunization |
80 |
78.24 |
16.73 |
20 |
99 |
MPI |
80 |
0.155 |
0.0835 |
0.02 |
0.422 |
Density |
80 |
1,239 |
7,724 |
0.400 |
69,341 |
Note: Control variables
are at district level.
Once the efficiency scores are estimated for each hospital, these are
aggregated at a regional (district) level. The reason for this aggregation is
the absence of latitude and longitude data for hospitals. The data on
district-level control variables have been taken from different sources. The
district-level data on some important variables are not available officially.
The district-level data on Human Development Index (HDI) and Multidimensional
Poverty Index (MPI) are taken from the literature [(Jamal, 2016) and (Naveed
& Ali, 2012)] respectively.
The MPI, used as a comprehensive indicator of poverty, is constructed
by utilizing the PSLM (Measurement, 2012-13) survey data, which covers 77500
households. The MPI score ranges from 0 to 1; the poverty level increases as we
move from 0 to 1. The average score of MPI is almost 16 percent. The authors have given equal weights (0.25)
in the measurement of MPI scores to all the four indicators i.e. education, health,
assets holdings and living conditions. Prenatal care and immunization are taken
as a proxy for health. Prenatal care is preventive healthcare which refers to
regular checkups of pregnant women before the childbirth. The average prenatal
care is almost 65 percent in all the districts. Immunization is a vaccination
for controlling life-threatening communicable diseases. The immunization is 78
percent, on average, in all the districts of Pakistan. Population density is
used as a proxy for demographic information. On average, the population density
of districts in the country is 1239 inhabitants per kilometer square (PSLM,
2011-12).
Empirical Specifications
This section is divided into two main parts. The first sub-section contains the empirical specifications for the measurement of technical efficiency. The specifications of the spatial models are provided in the second sub-section.
Efficiency Measurement
Efficiency is measured by examining the relationship between output (product of the health care system) and inputs (the resources used to produce that output). Initially, Farrell (Farrell, 1957) explained the concept ‘measures of efficiency’ and divided efficiency into two constituents; technical efficiency (produces the maximum output for a given level of inputs or employed the minimum resources to produce a fixed level of output) and allocative efficiency (The Allocative efficiency requires the information related to the relative prices of inputs and outputs. A firm is allocative efficient if it maximizes profit for a given cost or minimizes cost to produce a given level of output). Productive efficiency or economic efficiency is determined by combining the concepts of allocative efficiency and technical efficiency (O’Neill, Rauner, Heidenberger, & Kraus, 2008). The concept of technical efficiency in production was first developed by Farrell (Farrell, 1957) and was further technologically advanced by others (Boles, 1966; Charnes, Cooper, & Rhodes, 1978; Färe & Lovell, 1978).
The two principle methods which are widely used to measure efficiency, in the literature of health economics, are Stochastic Frontier Analysis (SFA) and Data Envelopment Analysis (Chilingerian & Sherman). (Aigner, Lovell, & Schmidt, 1977; Battese & Corra, 1977; Meeusen & van Den Broeck, 1977) proposed the method of SFA based on Farrell’s approach. The technical efficiency scores are measured by using Stochastic Frontier Analysis (SFA) production function taking inpatients and outpatients as dependent variables.
SFA is a parametric technique of efficiency analysis and it is based on the econometric regression model. It requires a functional form. It allows the random error along with the inefficiency term. One drawback of SFA, however, is that it allows only one output. The Stochastic Production Frontier of Cobb Douglas form is given below;
lny_i=?+ln?(x_i^')?_i+v_i-?_i
Where y_i represent the output of ith hospital; x_i^' is the vector of inputs of ith hospital and ?_irepresent the vector of parameters. v_i,?_i are two parts of error term with the assumptions that v_(i ) ~ iidN(0,?_v^2) , ?_i with exponential distribution, and ?_i and v_(i ) are independently distributed of each other and also of the regressors. The symmetric error term v_(i ) is the usual error term to allow for random factors like measurement errors, weather, strikes etc. The non-negative error term ?_i is the inefficiency component.
Spatial Analysis
To investigate whether the aggregated efficiency of the hospitals of a district is strategic complement or substitute of its neighboring district’s efficiency, we use the following relation,
? E?_i=f(E_j,X_i,?_i ) i=(1,……,I);j=(1…..,J) (1)
Where E_i is the average efficiency score of district i’s hospitals; E_j is the average efficiency of district j’s hospitals; X_i is the vector of control variables at district level (health, education, population etc.) and ?_i is random error. Global Moran’s I test is used to check the existence of spatial dependence of efficiency scores among the private hospitals of different districts. In the presence of spatial dependence, we estimate spatial cross-sectional lag and error models after controlling for the observable covariates. The spatial cross-sectional lag and error models are described in equations 2-4 as follows;
E_i=??_j??w_ij E_j ?+?^' X_i+?_i (2)
and
E_i=?^' X_i+?_i (3)
where
?_i=?w_ij+?_i (4)
Where E_j is the average efficiency of all hospitals in district j which is rival of average efficiency of hospitals in district i; w_ij is the weight matrix associated with the spatial interaction among hospitals, and X_i contains the covariates and ?_i is the random error. Equations 2-4 can be rewritten in matrix form,
E=?WE+X?+? (5)
and
E=X?+? (6)
?=?W+? (7)
Where W is the weight matrix composed of w_ijand spatial weights are generated by the inverse distance function.
w_ij={?(0 if i=j@d_ij^(-1) if d_ij?111km and i?j@0 if d_ij>111km and i?j)?
Where d_ijis the distance between two districts. In the current analysis, it is found that 111 km is the radius in which the hospitals in two different districts compete. In other words, districts that are within 111 km radius are assigned positive weights whereas zero weights are assigned to those that are beyond 111 km radius. WE is weighted average of efficiency of neighboring district and the weight matrix W is row standardized meaning that sum of all the elements of each row equals to one. ? (?) is a key coefficient and it confirms the existence of spatial autocorrelation when it is strictly positive and significant in the spatial lag (error) model. The spatial autocorrelation among the hospitals of different districts can exist due to (i) strategic interaction, ii. common unobserved characteristics of hospitals and, iii. observed or unobserved characteristics of neighboring districts.
Results and Discussion
This section is broadly divided into two main subsections following the objectives of the study. The first sub-section empirically estimates the technical efficiency for each hospital included in our analysis. The analysis of district-level spatial dependence in hospital efficiency is discussed in section 4.2.
Efficiency Measurement
The first step of our empirical analysis is to measure the efficiency of private hospitals without considering the prospective spatial interdependence between the units. The efficiency score lies between 0 and 1: a score of one represents that the hospital is fully efficient. The inefficiency is measured by the difference between the efficiency score of a hospital and the value one. The focus of the current study is an output-oriented model (The output-oriented technical efficiency is to obtain maximum output by utilizing a given set of inputs). That is, the highest possible level of output should be achieved with the given resources to reduce inefficiencies.
The findings of efficiency measurement, taking both inpatients and outpatient as combined output variables, reveal that not a single hospital lies on the production frontier and, therefore, none of the sample hospitals in our analysis is fully efficient. In relative terms, however, the most efficient hospitals are found to be Buner Medical School from Buner district and Malik Medical Complex from district Muzaffar Garh with efficiency scores of 0.73. This means that, even for these hospitals, 23 percent more output (inpatient and outpatient treatment) can be achieved with the same inputs. These two hospitals are Individual Proprietorship hospitals. On the other hand, the least efficient hospital is the Ramey Surgical Hospital and Maternity from district Bahawalpur.
Considering only the number of outpatients as an output variable in efficiency measurement, Manawar Hospital, with a score of 0.84, emerges as the most efficient hospital located in Faisalabad. The second most efficient hospitals, both run as individual proprietorships, are Alam Hospital from Gujrat and Fatima Medical Centre from Rajan Pur districts. When the number of inpatients is taken as the output variable in the measurement of technical efficiency, Yousaf Surgical Centre from Lodhran district and Turbat Medical Centre from Turbat surface as the most efficient health care facilities exhibiting 80 percent efficiency. Once again, these are individual proprietorships.
The overall average efficiency score of 749 private hospitals is 0.48. Hence, on average, the private hospitals are only 48 percent efficient and there is a tremendous room for improvement in this sector. The average efficiency scores across four provinces of Pakistan are presented in the Figure 1.
Figure 1
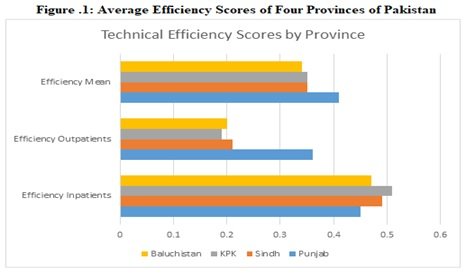
The majority of the hospitals included in the analysis are from Punjab (417), followed by Sindh (181), KPK (116) and Baluchistan (35). Punjab appears to be the most efficient province in treating outpatients. However, it becomes least efficient when it comes down to inpatient treatment. In the overall treatment of inpatients and outpatients, nonetheless, Punjab dominates all other provinces. KPK is most efficient in inpatient treatment and least efficient in outpatient treatment. Sindh secures the second position for the most efficient province in all three scenarios. Baluchistan illustrates a mixed picture for three cases.
Figure 2
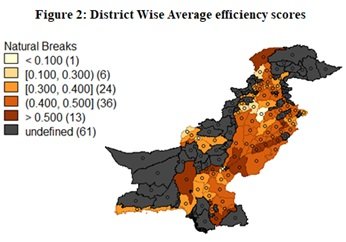
The aggregated findings at the district level, provided in Figure 2, show that none of the districts, from the 80 districts for which the data was available, is found to be fully efficient when both inpatients and outpatient are taken as output variables in the efficiency analysis. Most of the districts’ efficiency scores are found to be less than 50 percent. The efficiency score of only 13 districts is greater than 0.5. The average efficiency score is approximately 40 percent which is quite low. Matiari district, with an efficiency score of 0.64, comes out as the most efficient district. It is followed by Lodhran and Khuzdar with a score of 0.60 each. Killa Abdullah from Baluchistan province is the least efficient district for this measure of efficiency.
Figure 3 maps the district-wise efficiency using the number of outpatients as output variable in the efficiency scores calculations. The overall situation is almost similar. None of the district lies on the frontier. The efficiency scores of six districts are greater than 0.60 and the most efficient district turns out to be Kasur which is located in Punjab province. The efficiency score of 15 districts is less than 0.10 which is an alarming situation and calls for attention.
Figure 3
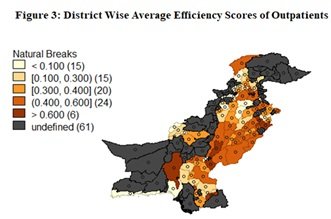
The efficiency scores are also measured by taking inpatients as an output variable. Inpatient services are provided by all the considered hospitals in the analysis. Inpatient care refers to a situation when a patient occupies a bed in the health care facility. In our analysis, there is a mix of big and small hospitals but all these hospitals provide inpatient facilities. The district-wise efficiency scores with inpatients as output variables are provided in Figure 4.
The maximum efficiency level is achieved by the district Lodhran with an efficiency score of 0.72, followed by districts D.I.Khan, Matiari and Upper Dir. Lakki Marwat and Killa Abdullah are the least efficient districts with efficiency scores below 0.20.
Figure 4
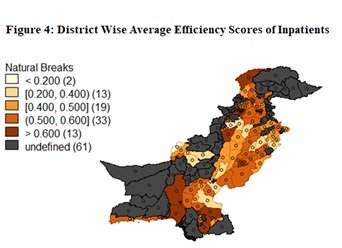
Spatial Analysis
The
existence of spatial autocorrelation inefficiency indicators and control
variables is examined using univariate Moran’s I test. For this purpose, the
inverse distance-based weight matrix is used. Moran’s I value is estimated
using weight matrices with two distances for sensitivity analysis. The values
of Moran’s I for efficiency indicators and all control variables, except
population density, are positive and highly significant for both distances at
conventional significance levels. It confirms the existence of spatial
dependence and spillover effects for almost all the variables. To capture this
spatial effect, we have applied spatial regression analysis by controlling for
health, population and poverty indicators. This analysis is done for three indicators
of efficiency to capture heterogeneous effects. Moreover, two types of weight
matrices are used for sensitivity analysis. The first is the inverse distance
weight matrix, whereas, the second is the contiguity weight matrix (The
contiguity weight matrix indicates that the districts share the boundaries. If
it shares the boundaries, then value 1 is assigned to that units and if not
then 0). For distance-based weight
matrices, the software packages take Euclidean distance (to convert the
Euclidean distance to the kilometers multiply the band by
Table 2. Univariate Moran’s I test for Spatial Autocorrelation
Variables |
Moran's I (d=1) |
Moran's I (d=2) |
Efficiency
mean |
0.178*** (0.08) |
0.124*** (0.054) |
Efficiency
inpatients |
0.149** (0.074) |
0.106*** (0.05) |
Efficiency
outpatients |
0.17** (0.08) |
0.173*** (0.048) |
Prenatal |
0.402*** (0.081) |
0.349*** (0.048) |
Immunization |
0.445*** (0.079) |
0.385*** (0.051) |
MPI |
0.456*** (0.073) |
0.393*** (0.053) |
Density |
0.011 (0.01) |
0.01 (0.005) |
Null Hypothesis: Values observed at one
location do not depend on values observed at neighboring locations. Standard errors
are in parentheses and *** indicates p<0.01, ** indicates p<0.05, and *
indicates p<0.1
We estimate OLS regression as well as two types of spatial models -
spatial lag and spatial error using both the weight matrices. While taking the
distance-based weight matrix, at the first distance four locations were
neighborless. However, when the distance was increased (band 2), then all the
districts have at least one neighbor. Results of all the
models while taking the average efficiency score, and efficiency scores
calculated taking outpatients and inpatients separately as outputs are provided
in Table 3.
Moran’s I values for the residuals for all the models in Panel A
(Table 3) are not significant showing the absence of the spatial dependence in
the case of average efficiency score. Nonetheless, our results of univariate
Moran’s I indicated the presence of spatial autocorrelation in all the
indicators. Hence, we disaggregated the analysis into two parts to identify
whether the spatial dependence exists in OPDs or in inpatient care for private
hospitals.
The Moran’s I values for all the models in Panel B, using both weight matrices,
are positive and highly significant showing strong spatial autocorrelation. The
Moran’s I value of OLS for d=1 and contiguity of order 1 is significant at 5
percent. When we increase the distance and take the neighbors in order 2, the
strength of spatial dependence further increases.
The coefficient of
spatial auto regression in spatial error model and the spatial lag model is
To check whether this behavior of private hospitals also prevails in
the case of inpatient care, we run these models using the efficiency score
obtained by employing inpatient as the dependent variable. These results are
reported in Panel C of Table 3.As evident from the results, the spatial
dependence does not exist in the case of inpatients because the value of
Moran’s I for residuals is not significant in any of these models. Moreover,
the values of Rho and Lambda are also insignificant. This suggests that the
behavior of private hospitals is not the same towards outpatients and
inpatients care. Competition exists for
OPDs in private hospitals but not for inpatient care. Therefore, the spillover
effects of efficiency have not been observed in the case of admitted patients
in private hospitals. An important reason behind this behavior could be the
size of the hospitals as most of the hospitals in the sample data are small.
Panel
A: Dependent Variable = Average Efficiency Score |
||||||||||||
Distance
Based Weight Matrix |
Contiguity
Based Weight Matrix |
|||||||||||
Band=1 |
Band=2 |
Contiguity
of Order 1 |
Contiguity
of Order 2 |
|||||||||
|
OLS |
Error |
Lag |
OLS |
Error |
Lag |
OLS |
Error |
Lag |
OLS |
Error |
Lag |
Moran’s I |
0.993 |
0.648 |
1.001 |
0.299 |
||||||||
Lambda |
0.195 |
0.042 |
0.112 |
-0.2 |
||||||||
Rho |
0.018 |
0.216 |
0.156 |
0.057 |
||||||||
Control |
Yes |
Yes |
Yes |
Yes |
Yes |
Yes |
Yes |
Yes |
Yes |
Yes |
Yes |
Yes |
Panel B: Dependent Variable = Outpatients |
||||||||||||
Moran’s I |
2.439** |
3.337*** |
2.104** |
3.533*** |
||||||||
Lambda |
0.429** |
0.474*** |
0.273* |
0.517** |
||||||||
Rho |
0.306** |
0.451*** |
0.211* |
0.53*** |
||||||||
Control |
Yes |
Yes |
Yes |
Yes |
Yes |
Yes |
Yes |
Yes |
Yes |
Yes |
Yes |
Yes |
Panel C: Dependent Variable = Inpatients |
||||||||||||
Moran’s I |
0.236 |
0.779 |
0.833 |
1.437 |
||||||||
Lambda |
-0.156 |
0.069 |
0.083 |
0.279 |
||||||||
Rho |
-0.131 |
0.145 |
0.079 |
0.231 |
||||||||
Control |
Yes |
Yes |
Yes |
Yes |
Yes |
Yes |
Yes |
Yes |
Yes |
Yes |
Yes |
Yes |
Table
3. Results of OLS,
Spatial Lag and Spatial Error Models for
Our analysis is based
on the data set of 749 hospitals which are mixed as big (no of beds>50) and
small hospitals (no of beds<50). Two different behaviors are experienced
from the same hospitals for two indicators; efficiency in OPDs and efficiency
in inpatients care. To explore this further, the given data-set of 749
hospitals is disaggregated into big and small hospitals.
The same exercise that has been done
previously is repeated for big and small hospitals separately to explore the
reasons behind two different behaviors for outpatients and inpatients care. Our
analysis started with the small hospitals and results are provided in Table 4.
We have estimated both the models’
spatial lag and spatial error models for robustness and found that both the
spatial coefficients, Lambda and Rho, are insignificant suggesting the absence
of spillover effects of efficiency for small private hospitals (Panel A: Table
4). To further explore the efficiency spillovers at the district level, we have
estimated the same models by taking the efficiency measured with inpatients and
outpatients as dependent variables separately (Panels B & C). The
estimation results in Table 4 show the existence of spatial dependence in the
case of outpatients. The values of the coefficient of spatial regression models
Table
4. Results of OLS,
Spatial Lag and Spatial Error Models for Small
Hospitals
Panel A: Dependent Variable =
Average Efficiency Score |
|||||||||||||
Distance Based Weight Matrix |
Contiguity Based Weight Matrix |
||||||||||||
Band=1 |
Band=2 |
Contiguity of Order 1 |
Contiguity of Order 2 |
||||||||||
|
OLS |
Error |
Lag |
OLS |
Error |
Lag |
OLS |
Error |
Lag |
OLS |
Error |
Lag |
|
Moran’s I |
1.22 |
0.86 |
1.166 |
1.021 |
|||||||||
Lambda |
0.218 |
0.099 |
0.166 |
0.118 |
|||||||||
Rho |
0.025 |
0.183 |
0.172 |
0.153 |
|||||||||
Control |
Yes |
Yes |
Yes |
Yes |
Yes |
Yes |
Yes |
Yes |
Yes |
Yes |
Yes |
Yes |
|
Panel B: Dependent Variable =
Outpatients |
|||||||||||||
Moran’s I |
2.435** |
0.129** |
2.748*** |
3.672*** |
|||||||||
Lambda |
0.50*** |
0.524*** |
0.315** |
0.469** |
|||||||||
Rho |
0.294** |
0.464*** |
0.272* |
0.491*** |
|||||||||
Control |
Yes |
Yes |
Yes |
Yes |
Yes |
Yes |
Yes |
Yes |
Yes |
Yes |
Yes |
Yes |
|
Panel C: Dependent Variable = Inpatients |
|||||||||||||
Moran’s I |
0.585 |
1.035 |
0.261 |
1.56 |
|||||||||
Lambda |
-0.119 |
0.132 |
-0.026 |
0.272 |
|||||||||
Rho |
-0.093 |
0.221 |
0.057 |
0.203 |
|||||||||
Control |
Yes |
Yes |
Yes |
Yes |
Yes |
Yes |
Yes |
Yes |
Yes |
Yes |
Yes |
Yes |
|
Note: Standard errors are in parentheses. *** p<0.01, **
p<0.05, * p<0.1.
Whether the profit-seeking
behavior of small private hospitals also exists for inpatient care is the next
logical question. This query is the basic motivation behind the estimation of
all the models using the efficiency obtained from inpatients as dependent
variable (Panel C). The findings reveal the absence of spatial autocorrelation
for inpatients care in small hospitals, as the values of Moran’s I are
insignificant in all the models with both the weight matrices. The values of
spatial regression coefficients
Table 5. Results
of OLS, Spatial Lag and Spatial Error Models for Big
Panel A:Dependent Variable =
Average Efficiency Score |
||||||||||||
Distance Based Weight Matrix |
Contiguity Based Weight Matrix |
|||||||||||
|
Band=1 |
Band=2 |
Contiguity of Order 1 |
Contiguity of Order 2 |
||||||||
|
OLS |
Error |
Lag |
OLS |
Error |
Lag |
OLS |
Error |
Lag |
OLS |
Error |
Lag |
Moran’s I |
-3.318 |
-1.429 |
-0.719 |
|||||||||
Lambda |
-0.064 |
0.054 |
0.059 |
-0.487 |
||||||||
Rho |
-0.088 |
-0.428** |
0.252 |
-0.277 |
||||||||
Control |
Yes |
Yes |
Yes |
Yes |
Yes |
Yes |
Yes |
Yes |
Yes |
Yes |
Yes |
Yes |
Panel B: Dependent Variable = Outpatients |
||||||||||||
Moran’s I |
-2.159 |
-1.03 |
-0.792 |
0.475 |
||||||||
Lambda |
-1.177 |
-1.37*** |
0.623** |
-0.012 |
||||||||
Rho |
-0.198 |
-0.790** |
-0.472 |
0.008 |
||||||||
Control |
Yes |
Yes |
Yes |
Yes |
Yes |
Yes |
Yes |
Yes |
Yes |
Yes |
Yes |
Yes |
Panel C: Dependent Variable = Inpatients |
||||||||||||
Moran’s I |
2.538** |
1.902* |
1.962** |
0.748 |
||||||||
Lambda |
0.094 |
0.051 |
0.315 |
0.129 |
||||||||
Rho |
-0.036 |
0.167 |
0.279 |
0.151 |
||||||||
Control |
Yes |
Yes |
Yes |
Yes |
Yes |
Yes |
Yes |
Yes |
Yes |
Yes |
Yes |
Yes |
Note: Standard errors are
in parentheses. *** p<0.01, ** p<0.05, * p<0.1
The analysis of big and small
hospitals has established that the spatial dependence exists for efficiency
when it is estimated with outpatients as output, whereas spillovers are absent
when the efficiency is estimated with inpatients in case of small hospitals.
Small hospitals are specialized in ambulatory care department and they compete
in this department with their neighbor regions to attract patients for
achieving maximum profit.
Concluding Remarks
This study explored the existence of spatial interaction among the private hospitals of Pakistan at the district level. First, the technical efficiency scores of all private hospitals are estimated using Stochastic Frontier Analysis. The existence of spatial autocorrelation is confirmed using Moran’s I test thereby verifying the evidence of regional spatial dependence. Subsequent spatial regression models, estimated using the maximum likelihood method, illustrated the presence of regional dependence for different cases.
The empirical findings present a bleak picture with regard to the overall efficiency scores for the private sector hospitals in the country. None of the hospitals is operating at full efficiency level. Hence, there is significant scope for improvement in efficiency for all the hospitals by increasing their output level given the same level of input resources. Efficiency scores are also estimated for two separate outputs - outpatients and inpatients for heterogeneous analysis.
Spatial regression analysis shows the existence of spatial dependence and positive efficiency spillovers at the regional level when outpatients is taken as a dependent variable. This confirms the existence of competition in OPDs of private hospitals. That is, the increase in efficiency in OPD of a hospital in one location induces the others to enhance their efficiency level as well. Subsequently, they can increase their profit levels by increasing their efficiency. However, spatial dependence has not been observed for inpatients. One may, therefore, conclude that the behavior of private hospitals varies regarding outpatients and inpatients to explore the reasons behind this behavior of hospitals, we have further disaggregated the analysis big and small hospitals.
The estimation results showed the spatial dependence in OPDs for small hospitals only. It could be because small hospitals constitute the major portion of the sample hospitals. The results for inpatients are, however, broadly inconsistent. One of the reasons behind the nonexistence of spillovers effect could be missing data as we have data for only 24 districts. Secondly, inpatient care in a hospital is conditional on the number of beds. Therefore, hospitals cannot increase the number of admitted patients beyond their capacity. According to the empirical literature, the size of the hospital has a real impact on the final health outcome (Giancotti et al., 2017). As the small hospitals specialized in ambulatory care, therefore hospitals compete with hospitals in their neighborhood to attract more patients to increase their profit levels.
These findings suggest that the government should focus on improving the efficiency of public hospitals to avoid private sector exploitation in the hospital sector. Moreover, policy interventions in terms of skill improvement or new technology adaptation will have substantial spillover effects on hospitals in the neighboring districts. Since private hospitals are not directly under the control of the government, this policy intervention can be made through a reduction in the import duties on new technology. The second stage analysis is done on district-level data due to data limitations. A better understanding of hospital behavior can be achieved with hospital-level data. This could be an important area for future research.
References
- Ahmed, S. (2011). Does economic geography matter for Pakistan? a spatial exploratory analysis of income and education inequalities. The Pakistan Development Review, 929-952.
- Aigner, D., Lovell, C. K., & Schmidt, P. (1977). Formulation and estimation of stochastic frontier production function models. Journal of econometrics, 6(1), 21-37.
- Augurzky, B., & Schmitz, H. (2010). Effizienz von Krankenhäusern in Deutschland im Zeitvergleich: Endbericht-November 2010. Retrieved from
- Banerjee, S., Carlin, B. P., & Gelfand, A. E. (2014). Hierarchical modeling and analysis for spatial data: Chapman and Hall/CRC.
- Battese, G. E., & Corra, G. S. (1977). Estimation of a production frontier model: with application to the pastoral zone of Eastern Australia. Australian Journal of Agricultural and Resource Economics, 21(3), 169-179.
- Berta, P., Martini, G., Moscone, F., & Vittadini, G. (2016). The association between asymmetric information, hospital competition and quality of healthcare: evidence from Italy. Journal of the Royal Statistical Society: Series A (Statistics in Society), 179(4), 907-926.
- Boles, J. N. (1966). Efficiency squared--Efficient computation of efficiency indexes. Paper presented at the Proceedings of the Annual Meeting (Western Farm Economics Association).
- Charnes, A., Cooper, W. W., & Rhodes, E. (1978). Measuring the efficiency of decision making units. European Journal of Operational Research, 2(6), 429-444.
- Chilingerian, J. A., & Sherman, H. D. (1997). DEA and primary care physician report cards: Deriving preferred practice cones from managed care service concepts and operating strategies. Annals of operations Research, 73, 35-66.
- Colla, C., Bynum, J., Austin, A., & Skinner, J. (2016). Hospital competition, quality, and expenditures in the us medicare population. Retrieved from
- Committee, O. C. (2012). Policy Roundtables: Competition in the Provision of Hospital Services: Paris: Organisation for Economic Co-operation and Development.
- Färe, R., & Lovell, C. K. (1978). Measuring the technical efficiency of production. Journal of Economic theory, 19(1), 150-162.
- Farrell, M. J. (1957). The measurement of productive efficiency. Journal of the Royal Statistical Society. Series A (General), 120(3), 253-290.
- Felder, S., & Tauchmann, H. (2009). Regional Differences in the Efficiency of Health Production: an Artefact of Spatial Dependence?
- Felder, S., & Tauchmann, H. (2013). Federal state differentials in the efficiency of health production in Germany: an artifact of spatial dependence? The European journal of health economics, 14(1), 21-39.
- Gaynor, M., Propper, C., & Seiler, S. (2016). Free to choose? Reform, choice, and consideration sets in the English National Health Service. American Economic Review, 106(11), 3521-3557.
Cite this article
-
APA : Bashir, S. (2020). Exploring the Spatial Interdependence in Efficiency of Private Hospitals in Pakistan. Global Social Sciences Review, V(I), 21-35. https://doi.org/10.31703/gssr.2020(V-I).03
-
CHICAGO : Bashir, Saima. 2020. "Exploring the Spatial Interdependence in Efficiency of Private Hospitals in Pakistan." Global Social Sciences Review, V (I): 21-35 doi: 10.31703/gssr.2020(V-I).03
-
HARVARD : BASHIR, S. 2020. Exploring the Spatial Interdependence in Efficiency of Private Hospitals in Pakistan. Global Social Sciences Review, V, 21-35.
-
MHRA : Bashir, Saima. 2020. "Exploring the Spatial Interdependence in Efficiency of Private Hospitals in Pakistan." Global Social Sciences Review, V: 21-35
-
MLA : Bashir, Saima. "Exploring the Spatial Interdependence in Efficiency of Private Hospitals in Pakistan." Global Social Sciences Review, V.I (2020): 21-35 Print.
-
OXFORD : Bashir, Saima (2020), "Exploring the Spatial Interdependence in Efficiency of Private Hospitals in Pakistan", Global Social Sciences Review, V (I), 21-35
-
TURABIAN : Bashir, Saima. "Exploring the Spatial Interdependence in Efficiency of Private Hospitals in Pakistan." Global Social Sciences Review V, no. I (2020): 21-35. https://doi.org/10.31703/gssr.2020(V-I).03